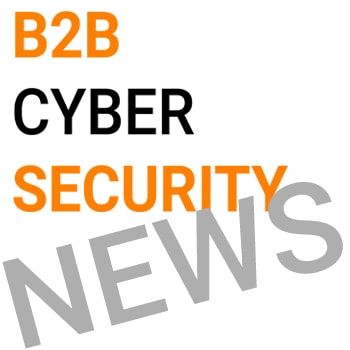
Many security teams still rely on static signatures to detect threats. They either rely on an intrusion detection system (IDS) for network analysis or on static behavioral detections based on endpoint logs.
But with more and more data, it becomes difficult to keep track and cover all sources and attack patterns with individual rules. To overcome these challenges, Exeon says machine learning (ML) algorithms help change the perspective of detection development. Anyone who uses ML can learn the normal state of communication, recognize deviations and evaluate them according to their dangerousness.
Exemplary areas of application of ML range from the detection of algorithms that generate domains to the analysis of traffic volume, the detection of command-and-control channels and the detection of internal propagation (lateral movement). In particular, IT security managers and SOC analysts who need to identify and defend against relevant threats can benefit from ML. Exeon itself uses machine learning algorithms and their baselining capabilities for its NDR (Network Detection & Response) platform.
Trained and untrained algorithms
Machine learning algorithms can be divided into two groups: trained and untrained algorithms. Both have advantages and some limitations in their application. The trained algorithms are trained in the lab to recognize known good and known bad features. Untrained algorithms use what is known as baselining, which means they learn the normal state of the infrastructure and can dynamically adapt their baseline to the company's environment. In the end this boils down to (network) statistics, calculation of probabilities, time analysis and clustering.
In combination, both groups of algorithms have great advantages, since on the one hand they draw on experience from the laboratory, industry and other customers (trained algorithms) and on the other hand learn dynamically in the company's own network (untrained algorithms) and on the basis of unknown, new ones to detect attacks.
Anomalies must be explained
When anomalies are detected, explanation and contextualization is paramount. Often security tools only report that the ML engine has detected something, but analysts don't know exactly what has been detected. Therefore, according to Exeon, it is important to provide the information that SOC analysts need; both with the precise naming of the anomaly and with context information directly on the anomaly. ExeonTrace uses trained and untrained algorithms, powerful visualizations and rich contextual information to efficiently and effectively support SOC analysts in detecting and resolving cyber incidents.
More at Exeon.com
About Exeon Analytics
Exeon Analytics AG is a Swiss cybertech company specializing in protecting IT and OT infrastructures through AI-driven security analytics. The Network Detection and Response (NDR) platform ExeonTrace offers companies the opportunity to monitor networks, immediately detect cyber threats and thus effectively protect their own company's IT landscape - quickly, reliably and completely software-based. The self-learning algorithms for early detection of cyber attacks were developed at ETH Zurich (Swiss Federal Institute of Technology Zurich) and are based on more than ten years of academic research. Exeon has received several awards, is internationally active and counts well-known companies such as PostFinance, V-Zug, SWISS International Airlines and the logistics group Planzer among its customers.