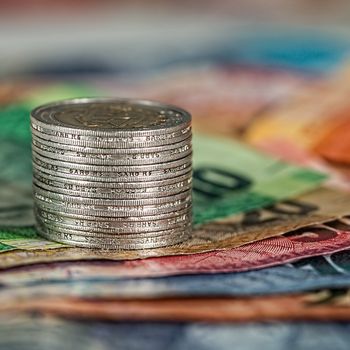
Companies are increasingly using artificial intelligence to optimize their operational efficiency and product innovation. A recent survey shows that 40 percent of companies surveyed want to increase their investments in AI technologies due to rapid advances in generative AI.
However, a downside to its increasing use is that AI - particularly generative AI - is computationally intensive and costs increase with the amount of data on which the AI models are trained. There are three main reasons why AI can quickly become a cost driver if left unchecked:
- AI consumed Additional resources: Running AI models and querying data requires large amounts of computing resources in the cloud, resulting in higher cloud costs.
- AI requires More computing power and storage space: Training AI data is resource intensive and costly due to increased computing power and storage space requirements.
- AI leads Frequent data transfers through: Since AI applications require frequent data transfers between edge devices and cloud providers, additional data transfer costs may be incurred.
If companies want to be successful with their AI implementation, they must understand and optimize the causes of rising costs. This can be done by adopting a solid FinOps strategy. FinOps is a public cloud management approach that aims to control the costs of cloud usage and where finance and DevOps meet. In addition, companies should consider the observability of AI.
Basics of AI observability
AI observability is the use of artificial intelligence to capture performance and cost data generated by various systems in an IT environment. Additionally, AI observability also provides IT teams with recommendations on how to mitigate these costs. How AI observability supports FinOps initiatives in the cloud by highlighting how AI adoption drives up costs due to increased usage of storage and compute resources. Because AI observability monitors resource usage across all phases of AI operations - from model training to inference to tracking model performance - companies can strike an optimal balance between the accuracy of their AI results and the efficient use of resources optimize operating costs.
Best practices for optimizing AI costs
- Cloud and edge-based approach to AI: Cloud-based AI enables companies to run AI in the cloud without having to worry about managing, deploying, or housing servers. Edge-based AI allows AI functions to run on edge devices such as smartphones, cameras or even sensors without the need to transfer the data to the cloud. Thus, by adopting a cloud and edge-based AI approach, IT teams can benefit from the flexibility, scalability and pay-per-use model of the cloud while reducing the latency, bandwidth and costs of sending AI data to reduce cloud-based processes.
- Containerization: Containerization makes it possible to package AI applications and dependencies into a single logical unit that can be easily deployed to any server with the required dependencies. Instead of statically adjusting the infrastructure to peak loads, companies can use a dynamically scalable container infrastructure for AI applications while optimizing costs.
- Continuous monitoring of AI model performance: Once a company trains AI models based on its data, it is important to continually monitor the quality and effectiveness of the algorithm. Monitoring AI models helps identify areas for improvement and “drift.” Over time, it can often be assumed that AI models will deviate from real conditions and therefore become less accurate. IT teams may need to adjust models to account for new data points. The decrease in predictive power as a result of changes in real environments that were not taken into account in the models must therefore be monitored.
- Optimization of AI models: This task goes hand in hand with the continuous monitoring of the models. It's about optimizing the accuracy, efficiency and reliability of a company's AI by using techniques such as data cleaning, model compression and data observability to ensure the precision and timeliness of AI results. Optimizing AI models can help save computing resources, storage space, bandwidth and energy costs.
- Proactive management of the AI lifecycle: The IT team's responsibilities typically include building, deploying, monitoring, and updating AI applications. AI lifecycle management ensures that AI applications are always functional, secure, compliant, and relevant using tools and procedures such as logging, auditing, debugging, and patching. Managing an AI lifecycle helps avoid technical issues, ethical dilemmas, legal issues, and business risks.
- Generative AI in conjunction with other technologies: Generative AI is a powerful tool. However, it only develops its full potential when combined with predictive and causal AI. Predictive AI uses machine learning to recognize patterns in past events and make predictions about future events. Causal AI makes it possible to determine the exact causes and effects of events or behaviors. Causal AI is critical to providing high-quality data to the algorithms that underlie generative AI. Composite AI brings together causal, generative and predictive AI to improve the collective insights of all three techniques. With Composite AI, the precision of causal AI meets the predictive capabilities of predictive AI to provide essential context for generative AI prompts.
The introduction of AI enables companies to be more efficient and innovative, but also carries the risk of escalating costs. Therefore, companies should proactively monitor and manage their AI models to ensure both the data accuracy and cost-effectiveness of their AI models. An overall strategy that incorporates FinOps and AI observability can help companies keep a close eye on the performance and costs of their systems.
More at Dynatrace.com
About Dynatrace Dynatrace ensures that software works perfectly worldwide. Our unified software intelligence platform combines broad and deep observability and continuous run-time application security with the most advanced AIOps to deliver answers and intelligent automation from data at remarkable scale. This enables organizations to modernize and automate cloud operations, deliver software faster and more securely, and ensure flawless digital experiences.
Matching articles on the topic