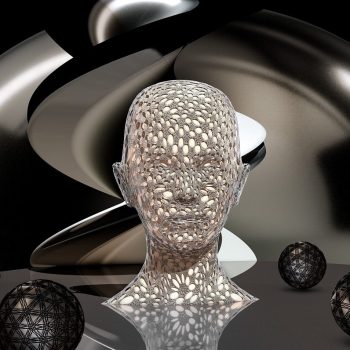
The constantly increasing number of successful cyber attacks demonstrates how often attackers achieve their goals despite modern prevention solutions. As a result, the focus is increasingly on technologies that serve to quickly discover ongoing attacks - NDR (Network Detection & Response). Artificial intelligence - AI - and machine learning - ML-based systems play a major role here.
However, since these terms are often mixed up and the topic of "AI & ML" is still a closed book for many companies, Andreas Riepen, Head of Central and Eastern Europe at Vectra AI, gets to the bottom of three fundamental questions.
Panacea or weapon aimed at companies?
One of the most pervasive myths stems directly from the extreme positions taken in the debate about the effectiveness of AI and ML as cybersecurity solutions. At one extreme is the argument that AI and ML are the panacea for all cybersecurity-related problems. At the other extreme is the argument that AI and ML play no role at all in cybersecurity. Unfortunately, the actual truth is far less headline-grabbing and less easily quoted by marketing departments. The fact of the matter is that AI and ML by themselves are not a panacea for your Security Operations Center (SOC). Forgoing AI and ML, however, would leave your SOC groping in the dark on a wide range of current and future attacks. It's easy to see why this is the case.
Solutions that do not leverage AI or ML cannot keep pace with the ever-changing landscape of attacker techniques and tools. Another (potentially more serious) problem is the fact that there are certain tasks that just cannot be done by humans alone. For example, humans are unable to look at a time series of encrypted network traffic flows to predict which of them might contain a hidden command and control channel. This type of task requires AI and ML solutions that go beyond human capabilities.
AI and ML solutions need to be better
On the other hand, solutions using only general AI and ML techniques, developed without security context and domain specificity, tend to simply look for statistical anomalies in an environment. These anomalies themselves are likely to be fairly common, although it is very unlikely that any of them are malignant. This leads to increased attention and operational costs, and distracts from the attackers' actual behaviors, which are often designed to appear harmless. Blindly trusting cyber security solutions based on generic AI and ML constructs is like trying to find a needle in a haystack by adding more hay first.
Another myth that continues to perpetuate is the notion that offensive AI poses the greatest threat to an environment. While there are new threats from the use of AI in cyberspace (e.g. using AI to generate credible human text for phishing campaigns and creating fakes, as we predicted a few years ago), the notion that AI-based systems are currently being used for end-to-end attacks is simply detached from reality. Anyone selling a security product to the customer on the premise that AI attackers are their primary threat, rather than determined and creative human attackers, may also have a workaround they're trying to sell you.
Human deficits in cybersecurity tasks
Organizations see AI as an opportunity to completely eliminate the human deficit in cybersecurity tasks. Is this realistic? The answer to this question depends in large part on how one interprets the word fix. The shortage of cybersecurity experts and the resulting risks are beyond question. This deficiency should cause deep and enduring concern among those involved in national security and economic planning. As our lives increasingly depend on digital, connected systems, we must face the fact that we create new attack surfaces much faster than we train experts to secure these systems.
With this in mind, it is necessary to point out that AI and ML can play a clear role in defusing the situation. There are ultimately two reasons why this is the case: First, AI and ML can be used to reproduce certain aspects of human behavior. Second, AI and ML can be used to go beyond what humans are capable of. In the first case, it is possible to automate parts of the workflow of security professionals. In the second case, AI and ML can be used to draw the experts' attention to the actual threats instead of focusing on superficial and harmless behaviors.
Ultimately, there is no way out of the shortage of cyber security experts. More people need to be trained in this area. Still, AI and ML will play a crucial role in helping experts find and remediate threats.
What should one know about the differences between AI and ML in security systems?
The distinction between AI and ML has probably become so fuzzy that trying to really separate the two terms isn't much use anymore. A much more important and fruitful avenue for companies is to ask themselves if the type of technology in a given solution is doing things that a human alone couldn't do. Does it save time for a human analyst? Or does it distract from the actual attacks the analyst hopes to uncover? Getting bogged down in whether or not it's AI or ML is akin to worrying about whether or not submarines float. Ultimately, it comes down to whether the solution works or not.
More at Vectra.ai
About Vectra Vectra is a leading provider of threat detection and response for hybrid and multi-cloud enterprises. The Vectra platform uses AI to quickly detect threats in the public cloud, identity and SaaS applications, and data centers. Only Vectra optimizes AI to recognize attacker methods - the TTPs (Tactics, Techniques and Processes) that underlie all attacks - rather than simply alerting on "different".